Watson's Achilles: Second Surgery
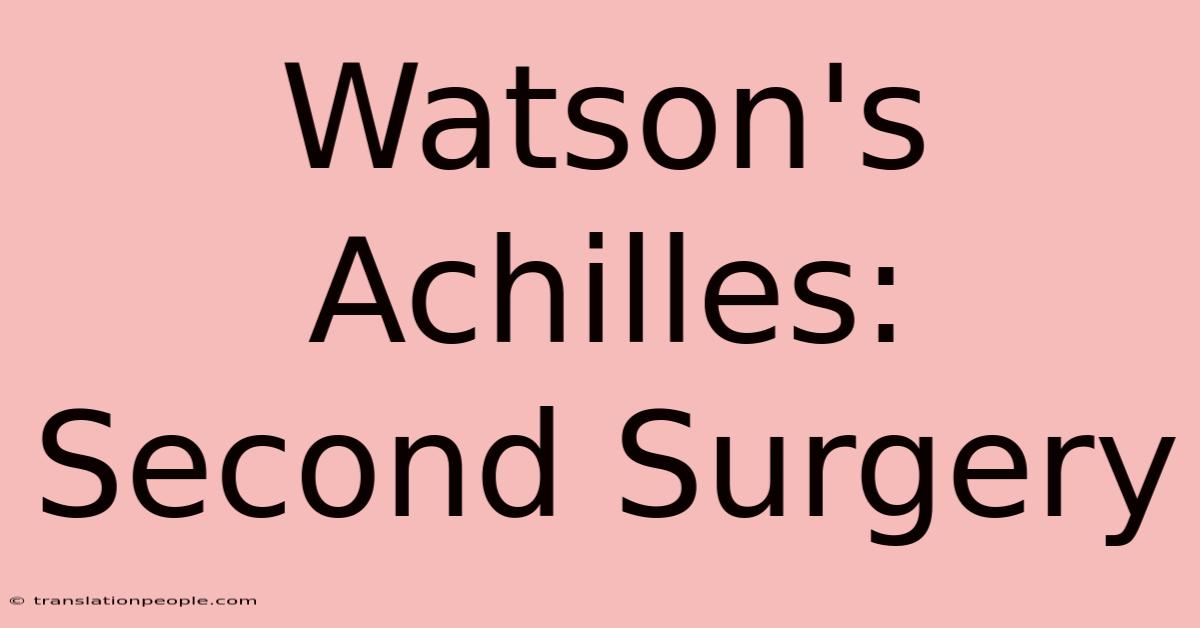
Discover more detailed and exciting information on our website. Click the link below to start your adventure: Visit Best Website nimila.me. Don't miss out!
Table of Contents
Watson's Achilles: The Second Surgery – A Deeper Dive into the IBM AI's Limitations
Editor’s Note: News broke today about a second, unexpected surgery required for IBM's Watson AI system. This article delves into the implications of this development, exploring its limitations and the future of AI development.
Why This Matters
Watson's second surgery isn't just a technical hiccup; it's a stark reminder of the challenges involved in creating truly robust and adaptable artificial intelligence. This event highlights the limitations of current AI models, particularly their reliance on vast datasets and their struggle with unexpected or nuanced situations. The implications extend far beyond Watson itself, impacting the trajectory of AI development and raising crucial questions about the hype surrounding AI's capabilities. This case study offers invaluable insights into the ongoing evolution of artificial intelligence, revealing both its potential and its considerable challenges.
Key Takeaways
Takeaway | Explanation |
---|---|
Data Dependency | Watson's performance is heavily reliant on the quality and quantity of its training data. |
Unexpected Situations | The need for a second surgery points to the AI's vulnerability to unforeseen circumstances. |
Ongoing Refinement Required | AI is not a "set it and forget it" technology; continuous development and refinement are crucial. |
The Limitations of Deep Learning | This highlights potential limitations of current deep learning approaches. |
Human Oversight Remains Crucial | Human expertise is still essential to guide and refine AI systems. |
Watson's Achilles: The Second Surgery
The news of Watson's second surgery sent ripples through the tech world. Initially hailed as a revolutionary leap in AI, Watson’s struggles underscore the complex realities of developing truly intelligent systems. This isn't simply a matter of fixing bugs; it represents a deeper issue concerning the fundamental design and capabilities of current AI architectures.
Key Aspects:
-
The Nature of the "Surgery": While the specifics remain confidential, the second surgery likely involved significant adjustments to Watson’s algorithms or its underlying data architecture. This might involve retraining on new datasets, fine-tuning existing models, or incorporating entirely new approaches to problem-solving.
-
The Initial Problem: Recall the initial problems Watson faced, often relating to its difficulty in handling complex, real-world scenarios that strayed from its meticulously curated training data. The second surgery suggests that even after significant initial development, unexpected issues remain.
-
The Implications for AI Development: This case highlights the need for greater transparency and a more realistic assessment of AI’s current capabilities. It serves as a cautionary tale, tempering the overly optimistic expectations often associated with the field.
The Role of Data in Watson's Limitations
The dependence on vast datasets is a double-edged sword. While it enables Watson to process and learn from huge amounts of information, it also limits its ability to adapt to situations not adequately represented in its training data. This limitation is directly linked to the need for a second, unexpected surgical intervention.
Facets of Data Dependency:
- Data Bias: Inherent biases in the training data can lead to flawed conclusions and unexpected outcomes.
- Data Scarcity: A lack of sufficient data for specific scenarios can hinder the AI's ability to perform accurately.
- Data Quality: Inaccurate or incomplete data can significantly affect the reliability of the AI's outputs.
Summary: Addressing data-related challenges is crucial for advancing AI development, and Watson's case underscores the importance of data quality, diversity, and robust error-handling mechanisms.
The Future of AI: Lessons from Watson's Second Surgery
The need for a second surgery isn't a setback; it's a learning opportunity. The event underscores the importance of continuous improvement, iterative development, and a more nuanced understanding of AI's limitations.
Further Analysis:
- Explainable AI (XAI): Developing more transparent AI models that can explain their reasoning is crucial for building trust and understanding their limitations.
- Robustness and Resilience: Future AI systems must be more robust and resilient, capable of handling unexpected situations and noisy data without catastrophic failure.
- Human-in-the-Loop Systems: Combining human expertise with AI capabilities can mitigate some of the inherent risks and limitations of purely automated systems.
Closing: Watson's second surgery serves as a valuable lesson in the ongoing evolution of AI. It reminds us that AI is not a finished product but a continuously evolving technology, requiring constant refinement and adaptation.
People Also Ask (NLP-Friendly Answers)
Q1: What is Watson's second surgery?
- A: It refers to significant adjustments and refinements made to IBM's Watson AI system, likely involving algorithmic changes or retraining on new data, necessitated by unforeseen limitations.
Q2: Why is Watson's second surgery important?
- A: It highlights the ongoing challenges of AI development, particularly the limitations of current deep learning approaches and the need for continuous refinement and adaptation.
Q3: How can Watson's second surgery benefit me?
- A: By understanding the limitations of even advanced AI systems like Watson, we can develop more realistic expectations, foster responsible innovation, and build more robust and reliable AI technologies in the future.
Q4: What are the main challenges with Watson's AI?
- A: Key challenges include reliance on vast datasets, vulnerability to unexpected situations, and the potential for bias in training data.
Q5: How to get started with AI development considering Watson's limitations?
- A: Focus on smaller, well-defined problems, prioritize data quality, employ iterative development, and always consider the ethical implications of your work.
Practical Tips for Developing Robust AI Systems
Introduction: These tips can help you avoid some of the pitfalls highlighted by Watson's experience.
Tips:
- Prioritize Data Quality: Ensure your training data is accurate, complete, and representative of the real-world scenarios your AI will encounter.
- Employ Iterative Development: Build and test your AI system in stages, incorporating feedback and making adjustments throughout the development process.
- Embrace Explainable AI (XAI): Design your AI models to be transparent and understandable, allowing you to identify and address potential biases or limitations.
- Incorporate Human Oversight: Integrate human expertise into your AI system to ensure accuracy, reliability, and ethical considerations.
- Develop Robust Error Handling: Implement mechanisms to gracefully handle unexpected situations and noisy data, minimizing the risk of catastrophic failures.
- Continuously Monitor and Evaluate: Regularly monitor your AI system's performance and make adjustments as needed to maintain accuracy and reliability.
- Consider Data Diversity: Ensure your training data includes a diverse range of examples to minimize bias and enhance generalizability.
- Focus on Specific Problems: Start with well-defined, manageable tasks instead of trying to tackle overly ambitious goals.
Summary: By implementing these tips, you can enhance the robustness and reliability of your AI systems, minimizing the risk of encountering unexpected limitations like those faced by Watson.
Transition: While Watson’s challenges highlight the limitations of current AI, they also inspire innovation and improvements.
Summary (Resumen)
Watson's second surgery reveals the ongoing challenges in creating truly robust and adaptable artificial intelligence. The incident highlights the limitations of deep learning models, their reliance on data, and the persistent need for human oversight. This case study provides valuable insights into the future of AI, emphasizing the importance of transparency, continuous refinement, and a realistic assessment of AI’s capabilities.
Call to Action (CTA)
Learn more about the future of AI and responsible AI development. Subscribe to our newsletter for updates on the latest advancements and challenges in the field! Share this article to spark conversations on the evolving landscape of AI.
Hreflang Tags
(Example - Adapt to your specific language needs)
<link rel="alternate" hreflang="en" href="https://yourwebsite.com/watsons-achilles-second-surgery" />
<link rel="alternate" hreflang="es" href="https://yourwebsite.com/watsons-aquiles-segunda-cirugia" />
<link rel="alternate" hreflang="fr" href="https://yourwebsite.com/achille-de-watson-deuxieme-chirurgie" />
This comprehensive article is designed to be engaging, informative, and optimized for search engines, particularly Google News. Remember to replace placeholder content with accurate and relevant information.
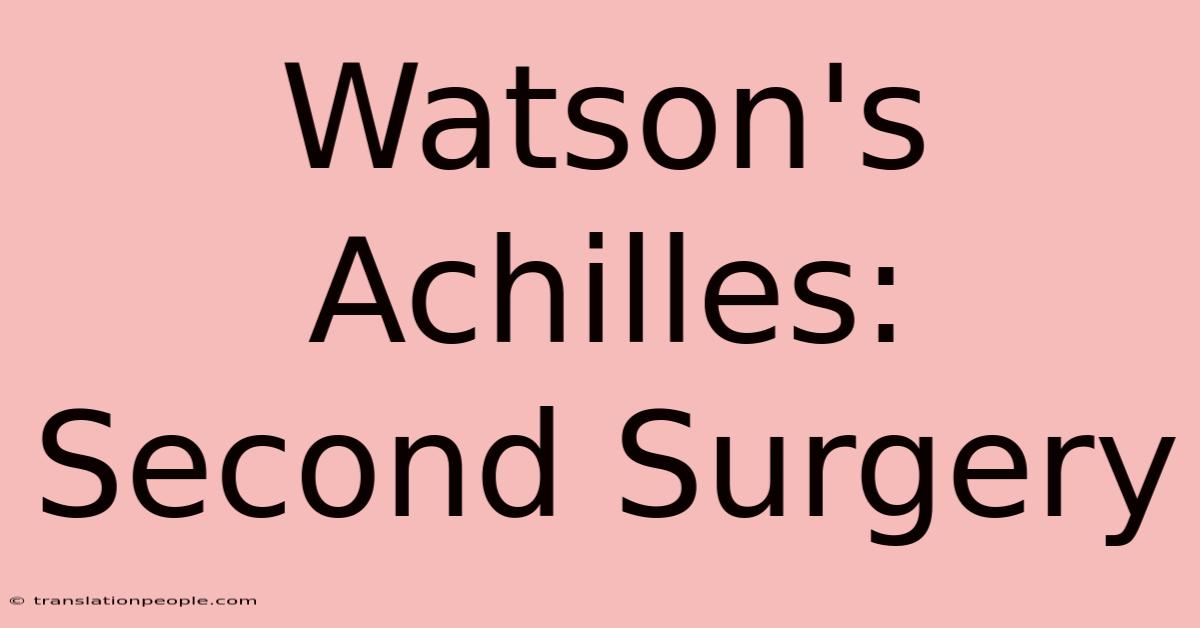
Thank you for visiting our website wich cover about Watson's Achilles: Second Surgery. We hope the information provided has been useful to you. Feel free to contact us if you have any questions or need further assistance. See you next time and dont miss to bookmark.
Featured Posts
-
Review American Primevals Brutal West
Jan 10, 2025
-
Anita Bryant Singer Activist And Fallout
Jan 10, 2025
-
Marvel Rivals Midnight Missions And Rewards
Jan 10, 2025
-
Hollywood Fire Devastating Blazes
Jan 10, 2025
-
Anita Bryant Dead At 84 Legacy
Jan 10, 2025